Table of contents :
Explainable AI (XAI): the imperative of algorithmic transparency
Discover how Explainable AI (XAI) is transforming business practices in response to the EU AI Act. This article explores the challenges of algorithmic transparency, explainability techniques, and sectoral applications.
Explainable AI (XAI) is radically transforming how organizations deploy their artificial intelligence systems by making their decisions understandable and justifiable. But how can we reconcile performance and transparency in increasingly complex models? How can we meet regulatory requirements while preserving innovation? This article guides you through the challenges, technologies, and best practices of explainable AI, now essential for any responsible AI strategy.
What is explainable AI (XAI)?
Explainable AI represents a set of methods and techniques aimed at making the decisions of artificial intelligence systems understandable by humans. Unlike traditional approaches where the internal workings of algorithms remain opaque, XAI reveals the "why" and "how" of algorithmic predictions.
Fundamental principles
Explainable AI is based on four essential pillars:
- Transparency: Ability to understand the internal workings of the model
- Interpretability: Possibility to explain decisions in understandable terms
- Justifiability: Demonstration of the reasoning behind each prediction
- Auditability: Complete traceability of the decision-making process
As a EESC report highlights: "Explainability is not just a technical requirement, but an ethical imperative that conditions the social acceptability of AI."
Key methods and techniques
Several technical approaches enable these explainability objectives:
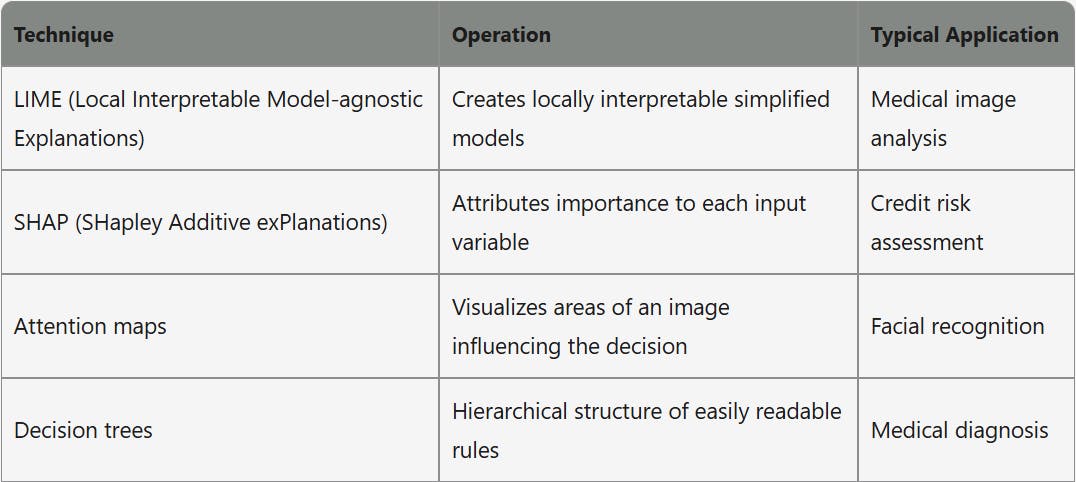
These methods transform complex models like deep neural networks into systems whose decisions can be explained and understood by various stakeholders.
The EU AI act and its implications for XAI
The European Union adopted in 2024 the world's first comprehensive regulatory framework dedicated to artificial intelligence, with strict requirements regarding the explainability of systems.
Risk-based approach
The EU AI Act categorizes AI systems according to four risk levels, each involving different obligations regarding explainability:
- Unacceptable risk: Prohibited systems (e.g., social scoring)
- High risk: Strict transparency and explainability requirements (e.g., recruitment, credit)
- Limited risk: Information obligations (e.g., chatbots)
- Minimal risk: No specific requirements
For high-risk systems, which concern many critical business applications, requirements include:
- Detailed documentation of training methods
- Complete traceability of decisions
- Ability to provide meaningful explanations to users
- Effective human oversight
Compliance timeline
Companies must adhere to a precise timeline to comply with these new requirements:
- June 2024: Entry into force of the EU AI Act
- December 2024: Application of prohibitions for unacceptable risk systems
- June 2025: Implementation of obligations for high-risk systems
- June 2026: Full application of all provisions
This progressive implementation gives companies the necessary time to adapt their AI systems, but requires rigorous planning.
Sectoral applications of explainable AI
The adoption of XAI is already profoundly transforming several key sectors.
Finance and insurance
In the financial sector, explainable AI addresses critical issues:
- Credit approval: Justification of loan denials in accordance with regulatory requirements
- Fraud detection: Explanation of alerts to reduce false positives
- Risk assessment: Transparency of actuarial models for regulators
A major European bank reduced its credit decision disputes by 30% by implementing SHAP models to explain each denial in a personalized way.
Health and medicine
The medical field, particularly sensitive, greatly benefits from XAI:
- Diagnostic assistance: Explanation of factors influencing disease predictions
- Medical imaging: Highlighting areas of interest in radiographs
- Therapeutic personalization: Justification of treatment recommendations
Google DeepMind has developed eye disease detection systems using saliency maps to highlight detected abnormalities, allowing ophthalmologists to understand and validate the proposed diagnoses.
Human resources
Recruitment and talent management are evolving with explainable AI:
- CV pre-selection: Transparency of filtering criteria
- Performance evaluation: Justification of automated ratings
- Attrition prediction: Explanation of identified risk factors
A study shows that candidates accept job rejections 42% more favorably when a clear and personalized explanation is provided.
Successful implementation: practical guide
Effectively deploying explainable AI requires a structured approach.
Assessment of explainability needs
The first step is to determine the required level of explainability:
Map your AI systems according to their impact:
- Criticality of decisions
- Applicable regulatory framework
- User expectations
- Sensitivity of processed data
Define audiences for explanations:
- End users (simple language)
- Business experts (specialized terminology)
- Regulators (technical compliance)
- Developers (technical diagnostics)
Establish explainability metrics:
- Comprehensibility (user tests)
- Fidelity (correspondence with the original model)
- Consistency (stability of explanations)
Adapted technological choices
Several technical approaches can be combined:
- Intrinsically interpretable models (decision trees, rules) for simple use cases
- Post-hoc methods (LIME, SHAP) for existing complex models
- Hybrid architectures combining performance and explainability
Open-source frameworks like AIX360 (IBM), InterpretML (Microsoft), or SHAP facilitate the implementation of these techniques without reinventing the wheel.
Governance and documentation
A solid governance framework is essential:
- Model registry documenting explainability choices
- Validation process for explanations by business experts
- Regular testing of explanation quality
- Comprehensive documentation for regulatory audits
Challenges and considerations
Despite its potential, explainable AI presents significant challenges.
Performance/explainability trade-off
One of the main challenges remains the balance between performance and transparency:
- Loss of accuracy: Simpler, more explainable models may sacrifice 8-12% accuracy
- Cognitive overload: Too many explanations can overwhelm users
- Computational cost: Some explainability methods significantly increase the necessary resources
Hybrid approaches, combining high-performance "black-box" models with explanation layers, are emerging as compromise solutions.
Persistent ethical issues
Explainability does not solve all ethical problems:
- Algorithmic bias: An explainable decision can still be biased
- Manipulation of explanations: Risk of misleading justifications
- False confidence: Simplistic explanations can induce excessive trust
A holistic approach to ethical AI must complement explainability efforts.
The future of explainable AI
The prospects for evolution in the short and medium term are promising.
Emerging trends
Several trends will shape the future of XAI:
- Multimodal explainability for systems simultaneously processing text, image, and sound
- Personalization of explanations according to the user's profile and needs
- Collaborative explainability involving humans and AI in constructing explanations
- Standardization of methods with the adoption of XAI-specific ISO standards
Sectoral perspectives
By 2026, according to analysts:
- 85% of financial applications will integrate native XAI functionalities
- 50% of medical systems will provide explanations adapted to patients
- 30% of companies will adopt "explainable by default" AI policies
Explainable AI is no longer an option but a strategic necessity in today's technological ecosystem. Beyond mere regulatory compliance, it represents a lever of trust and adoption for artificial intelligence systems.
For organizations, the challenge now is to integrate explainability from the design of AI systems, rather than as a superficial layer added afterward. This "explainability by design" approach is becoming the new standard of excellence in responsible AI.
In a world where trust becomes the most precious resource, explainable AI constitutes the essential bridge between algorithmic power and human acceptability. Companies that excel in this area will not only comply with regulations but will gain a decisive competitive advantage in the digital trust economy.
author
OSNI
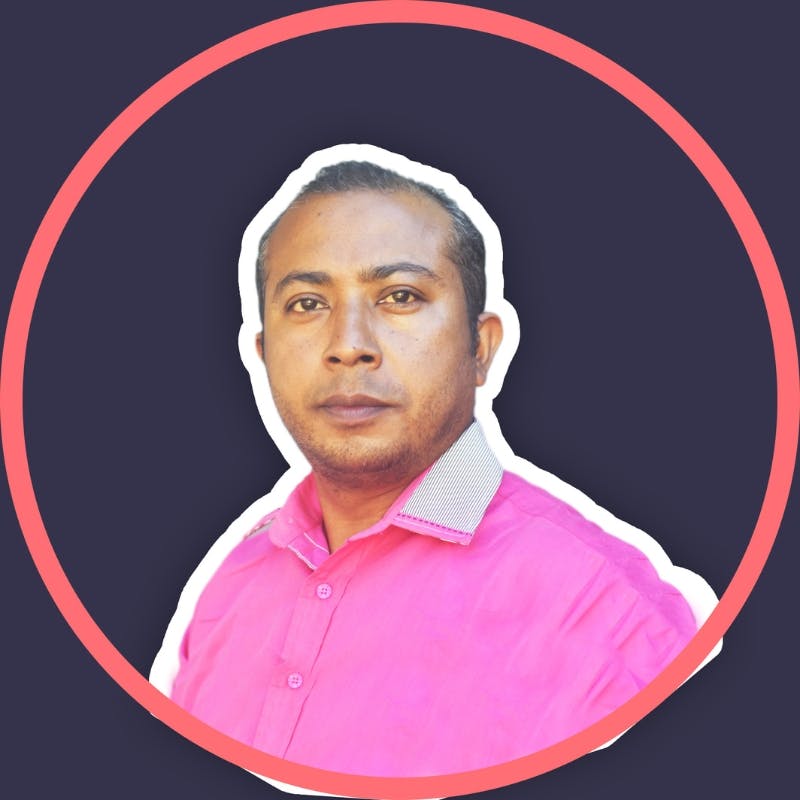
Published
March 20, 2025